Web of lies
We can combat misinformation with scepticism and content provenance technology
Imagine a world in which 95 per cent of images are real and 5 per cent are realistic fakes. And in this world, we assume all images are real by default.
Now imagine a world in which 5 per cent of images are real and 95 per cent are realistic fakes. Here, by default, we assume all images are fake.
Which world contains more misinformation? If we also assume that there is no way in either world to verify whether an image is real or fake, the answer is the first. This is because whether an image is deemed to be misinformation hinges — or should do — not on whether it is realistic but whether it is believed to be real. If nobody believes it, it isn’t misinformation, it’s just pixels. It could be art, social commentary, or nothing at all. By the same token, even if a fake image is technically unrealistic, if people are convinced by it, it should still be considered misinformation. This applies to disinformation — false information spread, often covertly, with the intention to deceive — as well.
The problem, of course, with the ultra-sceptical second hypothetical world, is that while it may not technically contain any misinformation, it doesn’t contain any information either. To avoid being duped by a fake image, we end up not being able to believe anything.
So while we can reduce the level of misinformation by increasing our level of scepticism, eventually we are left with nothing to believe, and no way to digitally communicate. In addition to the obvious harms of this — political unrest, educational challenges, disruption to almost every industry — consider also that incriminating photographic evidence would not exist, because there would always be reasonable doubt that images were real.
The solution to this is known as digital content provenance
But these worlds are not just hypothetical. Whereas we may once have lived in something like the first scenario, we are now far closer to the second than most of us are aware of. There have already been more images generated with AI than have been taken with cameras, and when more of these are indexable by search engines, the entire landscape of the internet will have changed. And in terms of realism, the days in which AI was incapable of (for example) generating believable hands are long gone. Media outlets promoting the idea that we can distinguish between AI and real images if we look carefully enough, are themselves disseminating untruths. That many creators of AI images don’t bother to run their program enough times to achieve five-fingered hands, is not evidence that AI is not capable of making them. Artificially generated images often have an overly perfect quality to them, but then so do filtered images on social media. Ultimately, debating how effective AI image generators can be is a waste of time, because they are are improving daily.
The solution to this is known as digital content provenance. In a world in which AI-generated images can be realistic, there is no way of knowing from an image itself whether it is real or fake, so we need accompanying trustworthy data which tells us its origin. Provenance and authenticity technology enables creators of real or fake images to log the source of the image when it is created, so that its status will never be in doubt.
So far, much of the discussion around how much of a misinformation problem AI-generated images pose has centred around how realistic they are. But the above thought experiment should illustrate that more significant factors in misinformation are 1) our automatic level of scepticism, and 2) whether effective content provenance technology exists and is widely adopted.
So what can we do? Firstly, increase our level of scepticism when it comes to what we see or hear online. Prepare for a world in which you might need to assume content is fake by default, unless it is verified. Don’t be complacent about generative AI’s abilities. Secondly, those who have the power to invest in new technology should direct their energies and capital towards content provenance technology and organisations. Businesses dealing with any kind of content — i.e. most of them — should also prioritise the adoption of content provenance tools.
Where to start? The Coalition for Content Provenance and Authenticity (C2PA) was founded in 2021, uniting Adobe’s Content Authenticity Initiative and Project Origin, a similar group led by the BBC, Microsoft, and The New York Times. Membership of C2PA is free.
Within the content provenance world, some tech is more useful than others for combating misinformation. Technology that tags an artificially generated image as artificial at the point of generation is limited in how useful it can be, because those who want to mislead people, or who don’t particularly care either way, will simply choose not to use such tools. Image watermarking is largely ineffective. Samsung’s new Galaxy S24 smartphone, which features generative AI tools, includes a watermark feature designed to highlight images created or altered with AI. However, the watermark can simply be removed using another Galaxy S24 editing tool.
Genuinely groundbreaking are developments that document the existence of real photographs at the moment of capture. For example, last year Reuters and Canon co-developed a content provenance photojournalism process and tested it in the field in Ukraine. Multiple technologies were used, for example Open Timestamps, which uses the Bitcoin network to link photograph metadata with the recorded data of mined blocks. The answers to the new problems posed by AI tend to lie in still newer technology, which is often complex and can be challenging to conceptualise. An extract from the Reuters-Canon project report summarises the process, and may require several reads:
In our proof of concept, a picture taken by our photojournalist is digitally signed by a Canon camera using a device-specific key at the point of capture and attached to the image, forming the first version of the picture. The authenticated picture’s pixels, GPS and other metadata, is sent to the Reuters system directly from the camera and registered onto a public blockchain as well as preserved on two cryptographic archives that use the Filecoin and Storj protocols.
Similar projects include Nikon’s recently-announced image provenance function in collaboration with Agence France-Presse.
The dangers of deepfakes are, of course, not limited to misleading content. In 2023 I worked with The Times and then BBC News to expose the widespread use of the AI image generators Midjourney and Stable Diffusion (released open source by UK-based Stability AI) to create sexualised images of children and child sexual abuse material. My investigations also found sexually explicit images of real women and celebrities being generated on a huge scale. This is reflected by a 2023 Home Security Heroes report that revealed 98 per cent of online deepfake videos to be pornographic. When specific individuals are targeted, the same report found, 99 per cent are women. Content provenance hardly helps us here, because the generation of such images constitutes abuse whether or not anyone believes they are real.
Fortunately, the harms of pornographic deepfakes are finally beginning to be acknowledged. When, in late January, AI-generated fake sexually explicit images of Taylor Swift went viral on Twitter, the White House described the situation as “very alarming”. Days later, the bipartisan Disrupt Explicit Forged Images and Non-Consensual Edits Act of 2024, was introduced in the Senate. The DEFIANCE Act would enable victims of sexually explicit deepfakes to sue the individuals who produce, receive or distribute the content.
Generative audio technology that can replicate real voices is particularly concerning
Returning to the misinformation challenge, video and audio content present variants of the same problem, and require parallel provenance and authenticity solutions. Text is different, because despite the changes wrought by generative text-based AI, written misinformation is hardly new. Generative audio technology that can replicate real voices is particularly concerning, as evidenced in the recent robocalls sent to Democrat voters in New Hampshire featuring fake recordings of Joe Biden urging listeners not to vote in January’s primary elections. This essay has focused on images rather than video or audio for clarity of argument, and because the challenges and remedies are parallel but not identical.
One positive and paradoxical outcome of content provenance solutions is that tools like these could actually herald a new era of truth in photojournalism. Because we can no longer afford to assume content reflects reality, and are being forced to develop better authenticating technology, we might end up with less, not more, fake news. The internet will become a sea of imaginings and hypotheticals, swirling around a bedrock of undoubtable, irrefutable truths, but with a change of perspective we could — bizarrely — have a greater grasp on reality than before.
But that era is not yet here: we are currently moving from a world in which most images are real, to one in which most images are fake, without the corresponding public awareness. Wider education regarding the effectiveness of AI tools (for example to reduce susceptibility to voice cloning scams, one solution to which is a known password among family members) is essential and urgent. Fortunately, the increasing development of content provenance technologies reflects a growing recognition that rather than directing our energies towards verifying falsehoods — an impossible task — we need to verify truths, which starts when an image is created. Investors take note.
Ultimately, blaming the strength of the AI tools themselves for the worsening misinformation crisis is a cop out: the solution is in our hands, and we must adapt.
Enjoying The Critic online? It's even better in print
Try five issues of Britain’s newest magazine for £10
Subscribe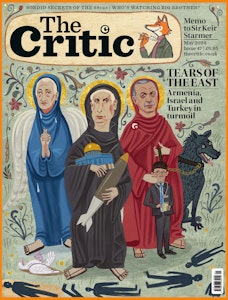